The State of European AI in 2025 | Insights from 150+ Tech Leaders
In conversations with over 150+ tech executives across Europe in the last 6 months, we’ve uncovered revealing patterns in how European companies are approaching AI adoption. These insights, gathered from CEOs, CTOs, CPOs, COOs and innovation leaders across multiple tech sectors, provide a ground-level view of the challenges, strategies, and opportunities shaping Europe’s AI transformation and form the basis for this report.
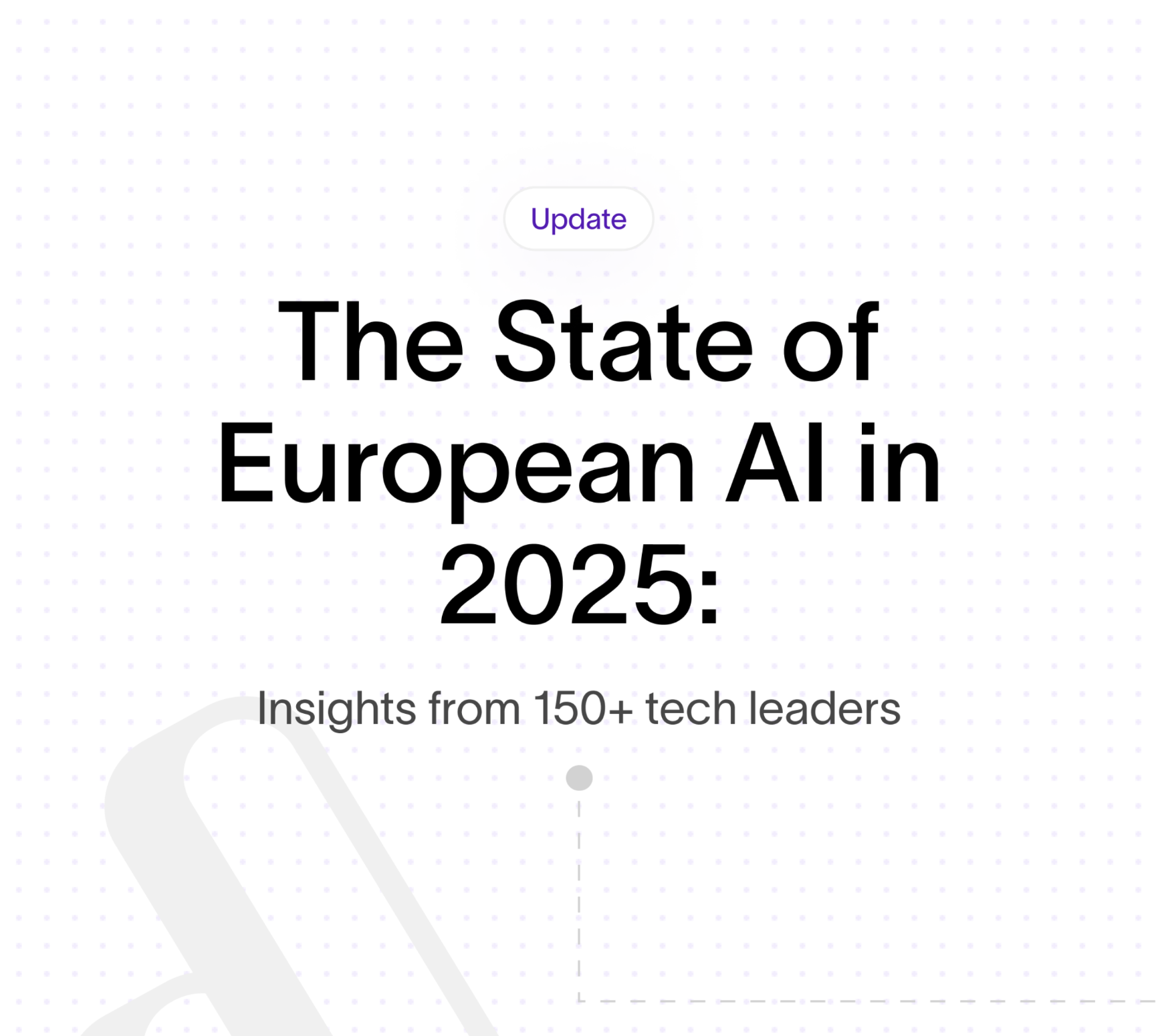
Executive Summary
The State of European AI in 2025 | Insights from 150+ Tech Leaders
In conversations with over 150+ tech executives across Europe in the last 6 months, we’ve uncovered revealing patterns in how European companies are approaching AI adoption. These insights, gathered from CEOs, CTOs, CPOs, COOs and innovation leaders across multiple tech sectors, provide a ground-level view of the challenges, strategies, and opportunities shaping Europe’s AI transformation and form the basis for this report.
—--
European SaaS companies are at a pivotal moment when it comes to AI adoption. While 74% of these SaaS businesses have initiated AI projects, only 14% have reached full production and are driving measurable revenue or cost savings.
This gap between enthusiasm and execution can be explained by European’s lagging eco-system around AI, regulatory complexities, data siloes, and an ongoing talent shortage. Yet the opportunity is immense: AI model costs have dropped 75% over the past two years, while performance has improved by 50%—enabling both internal productivity boosts and customer-facing innovations.
Despite lagging behind the US by >12-months in AI adoption, Europe has some particular advantages, such as strong data protection frameworks and a reputation for ethical and sustainable tech. When harnessed effectively, these strengths could offer a unique differentiator in regulated sectors like healthcare and finance, but will prove challenging in faster moving sectors.
The road ahead to keep up requires a focused approach: define a clear strategy, pick a small set of high-impact use cases, build dedicated internal resources around AI, and prioritize data governance, availability and privacy from day one. In 2025, European SaaS leaders who are able to adapt to the new possibilities, while staying compliant with EU regulations and concerns of clients, will be reshaping how software is delivered, monetized, and experienced across the continent.
While possible, a real shift and focus on AI is needed to catch up with the US and China. This is not only a ‘state of’ report. It’s also a call to arms for EU tech companies to start moving. Fast.
Introduction: the global AI race intensifies
The AI hype is everywhere—from AI-powered fridges to AI-generated flavors of ice cream—yet for many European SaaS firms, the real question remains, “Where’s the payoff?”
Although ChatGPT and image-generation tools have captivated headlines, in practice, only a minority of European tech companies have transformed AI pilots into tangible business results. Most are still wrestling with where AI fits in their roadmaps, how to handle privacy concerns, and how to train their teams.
Meanwhile, the global AI race is intensifying. Tech giants like Microsoft and Google invest billions into infrastructure, while startups like OpenAI and Anthropic raise record funds. Faced with a >12-month lag behind the US, European SaaS can no longer ignore AI as a passing fad—it’s shaping up to be a baseline capability rather than a fringe experiment.
In the report that follows, we synthesize insights from 150+ product and tech leaders in Europe, revealing how companies are navigating these challenges and what steps they must take to compete effectively.
Whether you view AI as a modest efficiency booster or a disruptive force poised to redefine software, one thing is clear: the clock is ticking for European SaaS to embrace AI or risk falling further behind their US and, soon, their Chinese counterparts.
1. Europe’s AI landscape in 2024
The implementation gap
Insights in European companies endeavours around AI reveal that:
- 74% of companies have begun some sort of AI project.
- 26% still have no AI adoption at all.
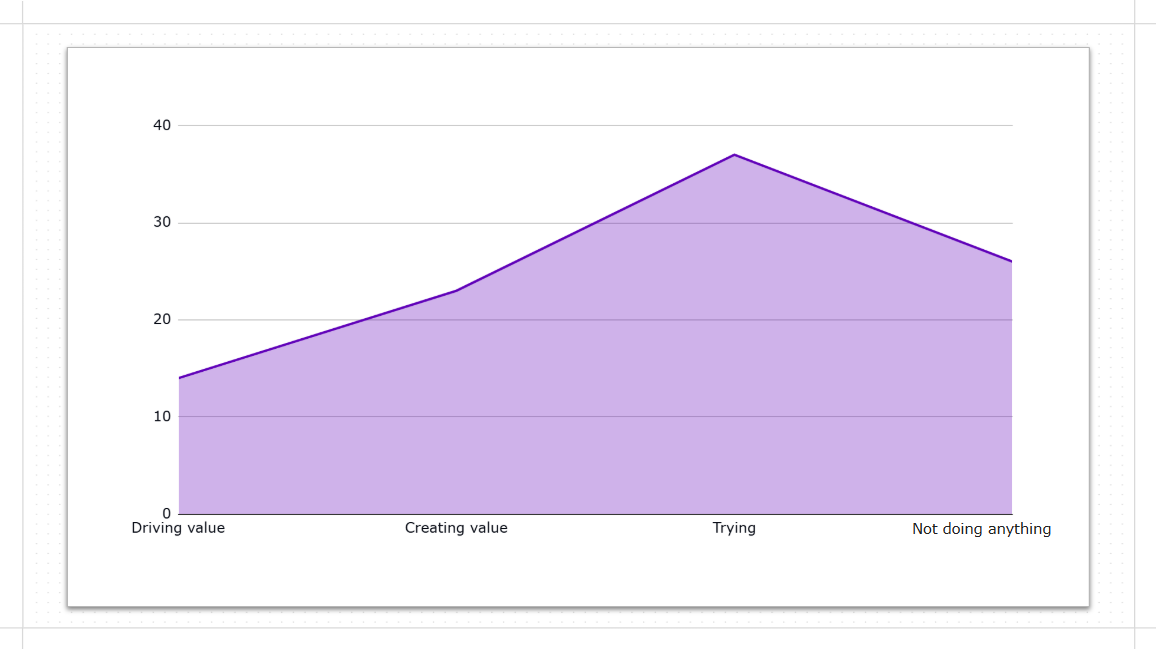
- 37% remain in the “trying or experimental” phase (pilots, proofs-of-concept, trying tools).
- 23% have ‘a’ AI in production (this can be both in their product or in their internal operation)
- 14% is actively driving value through AI with features in their product and/or with AI tools significantly improving the quality of their work or reducing time spend
This underscores widespread AI awareness but limited success in the execution. Many firms remain unsure about how to integrate AI tools, acquire or train talent, and/or how to tackle privacy regulations.

Growing market, shifting strategies
Fueled by a 75% cost drop in AI models—and a 50% performance uptick—Europe’s spending on SaaS AI is expected to exceed €14 billion by the end of 2025. Emerging startups like Mistral prove Europe can compete globally when capital and vision align.
This market evolution is driving established players like Klarna, Notion, Intercom and Miro to shift beyond simple "magic wand" demos to embed AI into customer-facing workflows that deliver sustained business value.
A key enabler of this transition is retrieval-augmented generation (RAG), which allows companies to cost-effectively connect AI applications to their proprietary data and documents. By grounding AI responses in domain-specific knowledge, organizations can deliver more accurate and relevant solutions for their industry needs.
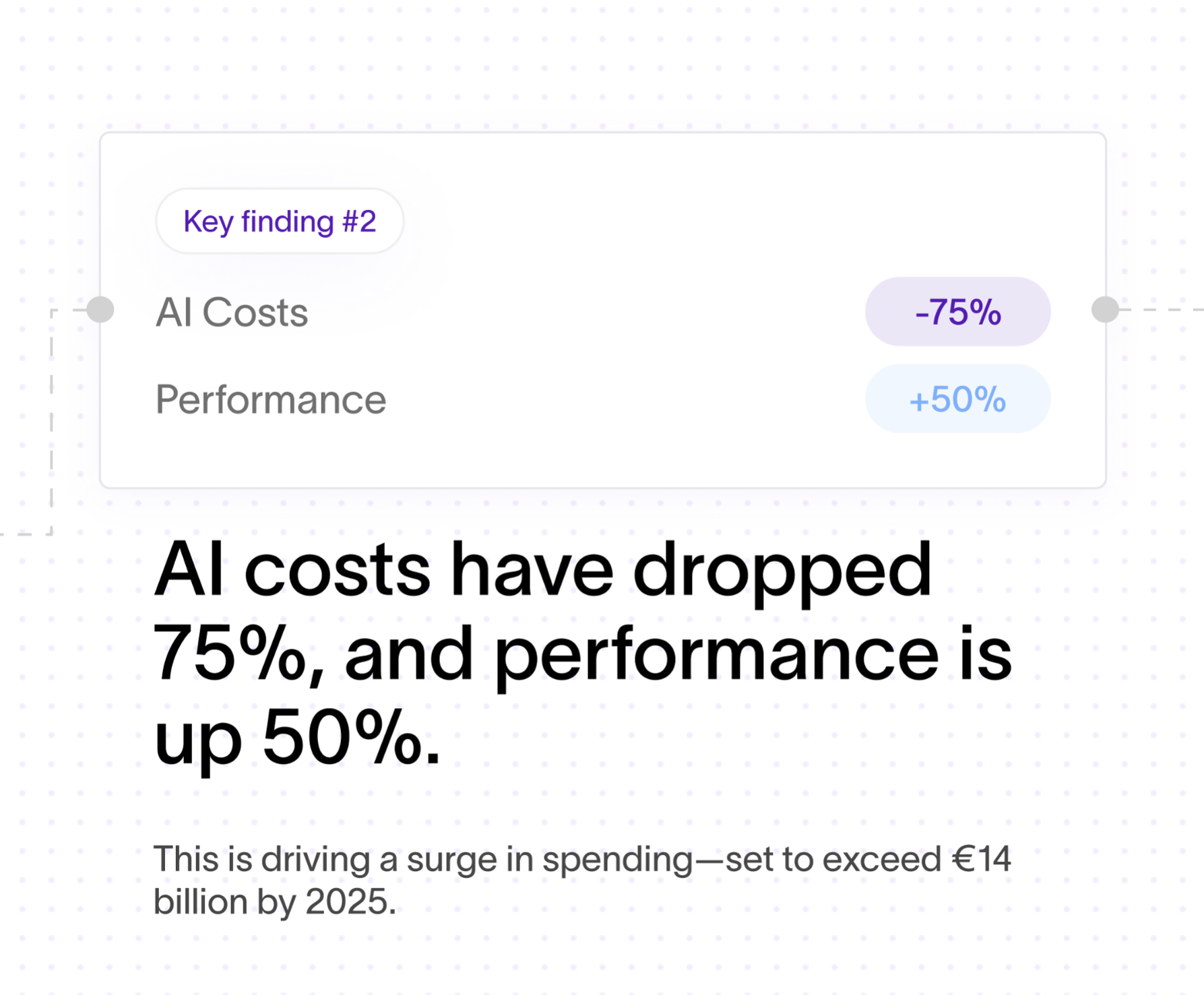
Sector snapshots
When we look at AI adoption across Europe, we see that there are huge differences between industries. This variance shows that there are fundamental differences in market pressures, regulatory environments, and customer expectations.
The sprint leaders: drive value fast
Marketing, support, and edtech companies find themselves in an “adapt or perish” moment. In these customer-facing sectors, AI isn’t just an advantage—it's becoming table stakes. Companies are rapidly deploying.
These sectors are emerging as AI innovation laboratories. Companies are moving beyond basic chatbots to develop sophisticated AI agents that can handle complex customer interactions, generate compelling content, and even proactively identify customer needs before they arise.
Healthcare & financial services: While bound by strict compliance requirements, these sectors are turning regulation into opportunity more and more. Banks are pioneering AI-powered fraud detection systems that respect GDPR (!), while healthcare providers are developing compliant AI diagnostics tools that could set global standards.
E-commerce: Leading the charge in practical AI applications, these companies are revolutionizing the shopping experience. From AI-powered personal shoppers to inventory systems that predict trends before they happen, retail is showing how AI can directly impact the bottom line.
The strategic laggers: evaluate and wait
Manufacturing and government sectors are taking a more calculated approach.
Manufacturing & logistics: Rather than rushing to adopt customer-facing AI, these industries are focusing on high-impact, low-risk applications. Predictive maintenance systems are already delivering ROI by preventing costly equipment failures, while AI-optimized scheduling is quietly revolutionizing supply chain efficiency.
Government & public sector: Public sector organizations remain cautious AI adopters, primarily due to procurement regulations, security requirements, and legacy system dependencies.
While some agencies are testing AI for document processing and basic customer service automation, most government bodies are still in the evaluation phase, watching private sector implementations before making significant investments. This wait-and-see approach, while slower, allows them to adopt proven solutions rather than cutting-edge experiments.
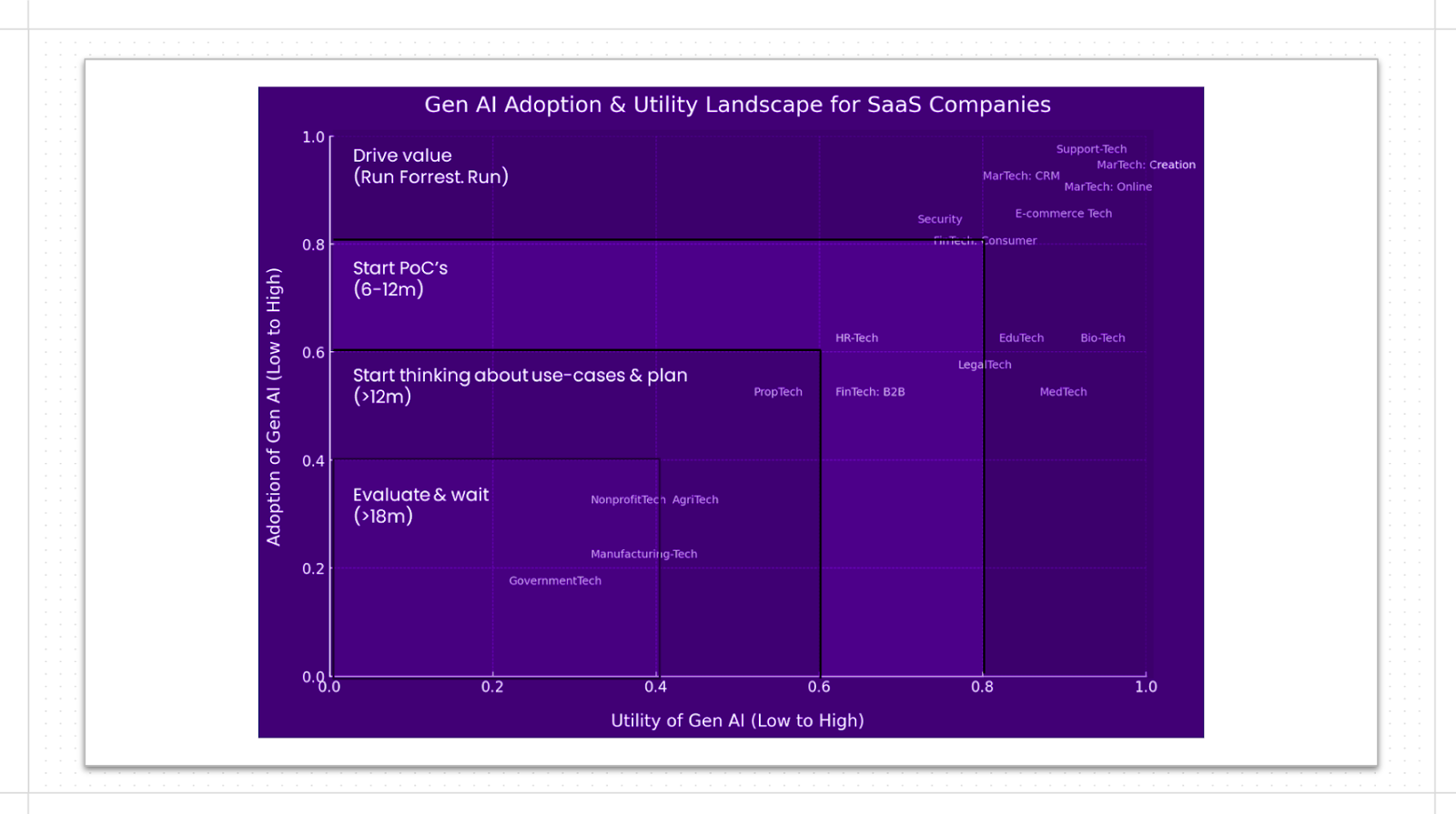
- Healthcare & financial services: Compliance-first approach makes AI both a hurdle and an opportunity.
- Retail & e-commerce: Focus on personalization, inventory optimization, and automated customer support.
- Manufacturing & logistics: Using AI mainly for back-office improvements (predictive maintenance, scheduling).
- Marketing & support tech: Leading in user-facing AI, harnessing chatbots, content generation, and early-stage AI agents.
Key trends to watch
European tech leaders see several key trends that are emerging that could reshape the industry's future.
- Regulation as differentiation: Companies embrace strict data governance, privacy controls, and ethical safeguards that are vital for global enterprise clients. Regulation and governance can also be seen as opportunities, not barriers
- Multi-vendor or hybrid models: Firms combine solutions from OpenAI, Anthropic, Gemini, and open-source frameworks to balance cost, control, and performance.
- Emergence of AI agents: Simple chatbots are evolving into autonomous agents that tackle sales, support, and DevOps tasks—12% adoption now, expected to triple in 2025.
- Services-as-a-Software: A shift from offering tools to delivering complete outcomes (e.g., fully automated workflows) signals a new era for SaaS.
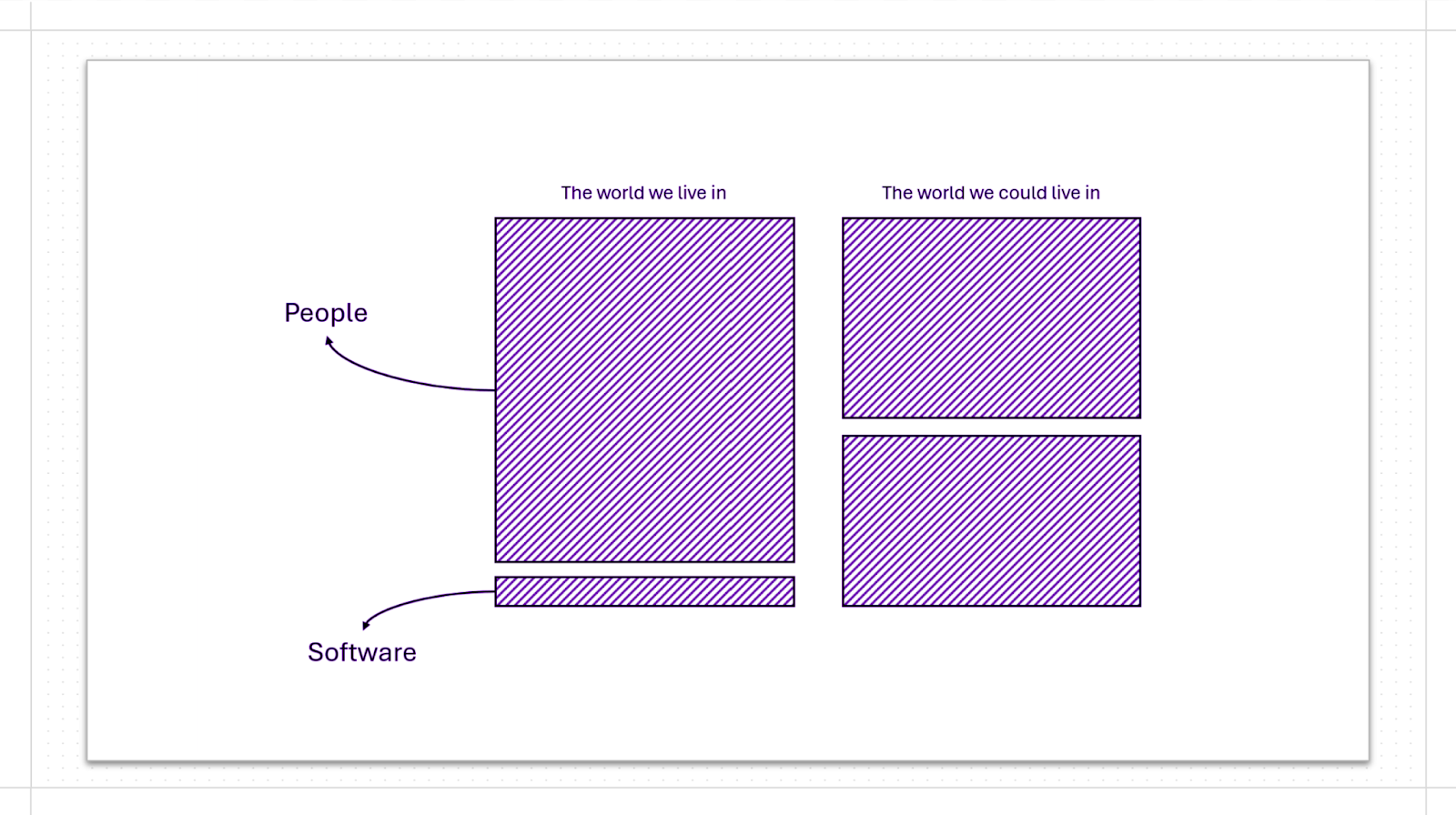
Overall, despite lagging behind the US, European SaaS companies are finding creative ways to leverage AI—particularly in heavily regulated sectors. Europe’s cautious but committed approach could become a strategic advantage in the years ahead.
LLM market share shift across models
We see a clear shift in market share split across LLMs and expect this trend to continue into 2025, with an expected rise of LLMs originating in China, as well.
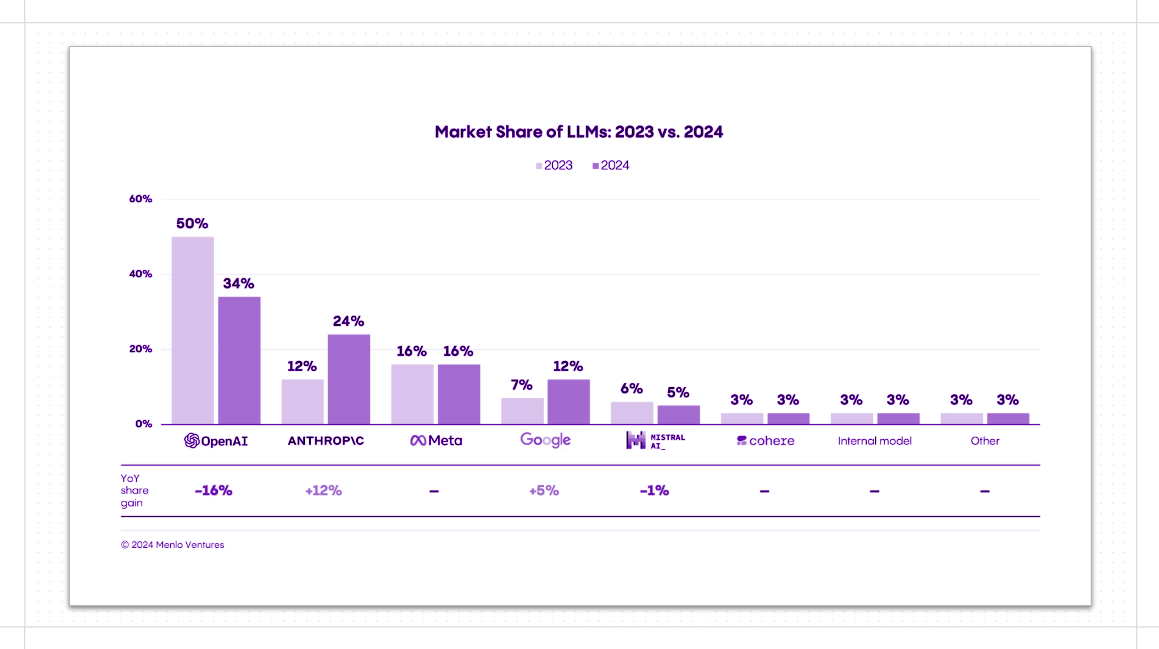
2. Research findings: insights from 150 European tech leaders
From innovation to core business
Companies are moving AI initiatives from innovation budgets into core operations, with 40% of AI projects now funded through standard budgets rather than traditional R&D. Early adopters now view AI capabilities as essential product features rather than experimental projects, using them to drive revenue and cut costs.
Implementation timelines & resource allocation
Companies successfully moving beyond pilots typically:
- Launch AI features in 4–6 months (down from 12–18)
- Dedicate 20–30% of their general technical resources to AI
- Allocate 15–25% of their overall core product development capacity to AI
These organizations begin with small and focused teams—often consisting of a product manager, developers, and possibly one data scientist—before they are scaling up.
Evolving investment patterns
Leading “AI-forward” companies allocate 18–25% of tech budgets to AI, which is then being distributed across:
- 30% Development (features, model integration)
- 25% Data Infrastructure (storage, processing)
- 20% Team Training (upskilling existing engineers)
- 15% External Tools (vendor platforms, APIs)
- 10% R&D (new proofs-of-concept)
Most prefer a hybrid approach—using off-the-shelf solutions (translation, sentiment analysis) while building proprietary components that are used for competitive differentiation.
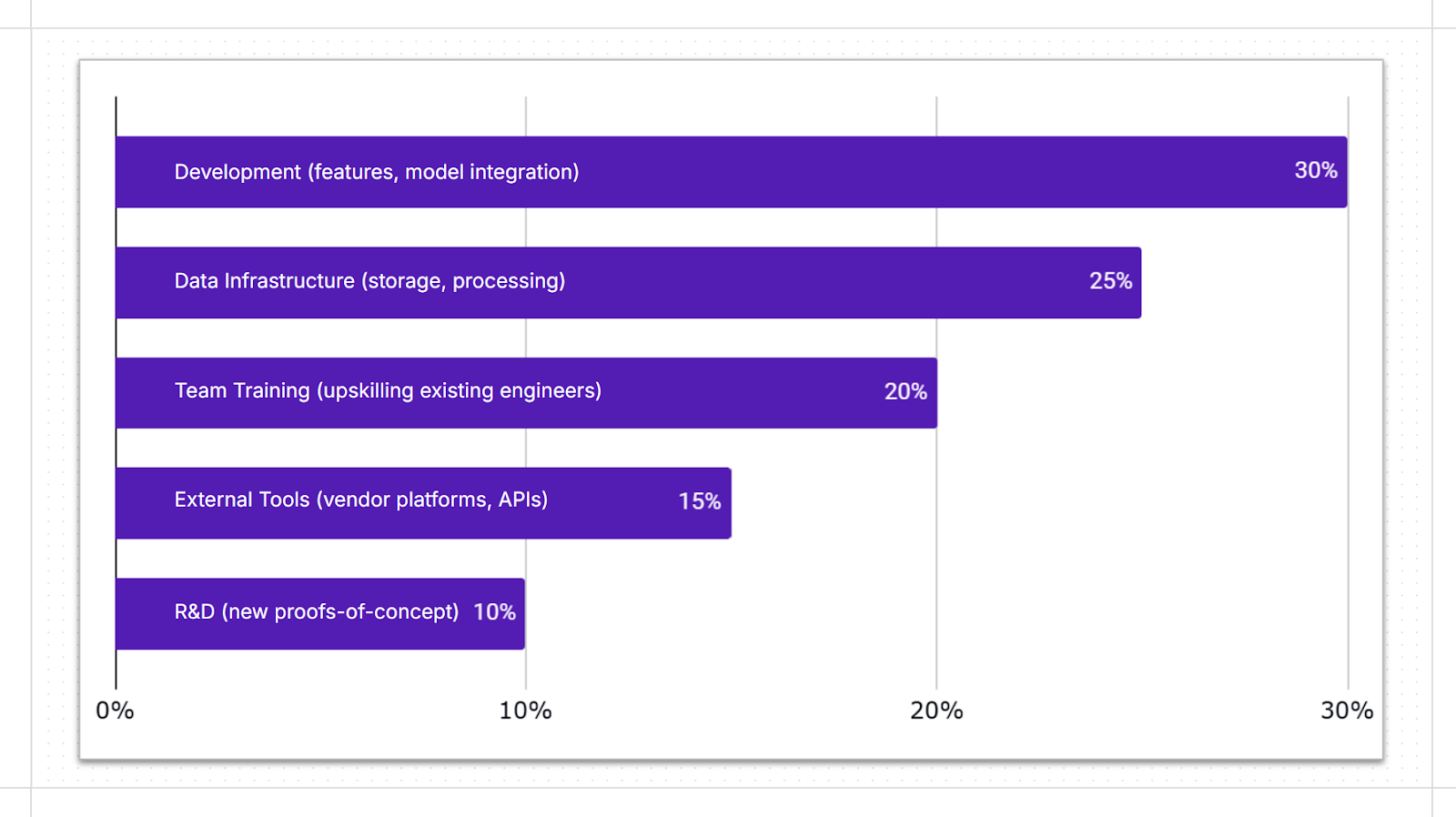
Departmental investment patterns
The distribution of generative AI spending across departments reveals where organizations are finding the most immediate value. Technical departments command nearly half of all AI investments:
- IT leads with 22% of spending, focusing on infrastructure and internal tools
- Product and Engineering follows at 19%, prioritizing AI-enhanced features
- Data Science represents 8%, concentrating on model development and analytics
Customer-facing functions also show significant investment:
- Customer Support at 9%, implementing AI for service automation and efficiency
- Sales operations claim 8% of spending, leveraging AI for lead generation and engagement
- Marketing allocates 7% to AI-powered content and campaign optimization
Back-office operations demonstrate balanced investment:
- Both HR and Finance/Accounting maintain 7% each, focusing on process automation
- Design teams claim 6% of spending, using AI for creative workflows
- Legal departments represent 3% of investment, primarily in document analysis and compliance
This distribution suggests organizations are prioritizing technical foundation-building while simultaneously pursuing customer experience improvements and operational efficiency. The relatively balanced allocation across departments also indicates AI's broad utility across the enterprise, rather than being confined to purely technical applications.

Early wins & key metrics:
The data from successful AI implementations tells a compelling story of transformation across key business functions. Organizations that have effectively integrated AI are witnessing serious improvements in operational efficiency and market responsiveness. These results aren’t just incremental gains—they represent fundamental shifts in how businesses operate and compete.
Successful AI adopters report:
Development velocity: Teams are reporting 35% faster development cycles through AI-assisted coding and automated quality assurance, enabling them to ship features at unprecedented speeds
Deployment efficiency: A remarkable 40% reduction in feature deployment times, powered by AI-optimized testing and release processes
Customer experience: Support teams are achieving 45% greater efficiency through AI-augmented response systems and predictive issue resolution
Content operations: Marketing and product teams are experiencing 50% acceleration in content creation, from documentation to marketing materials
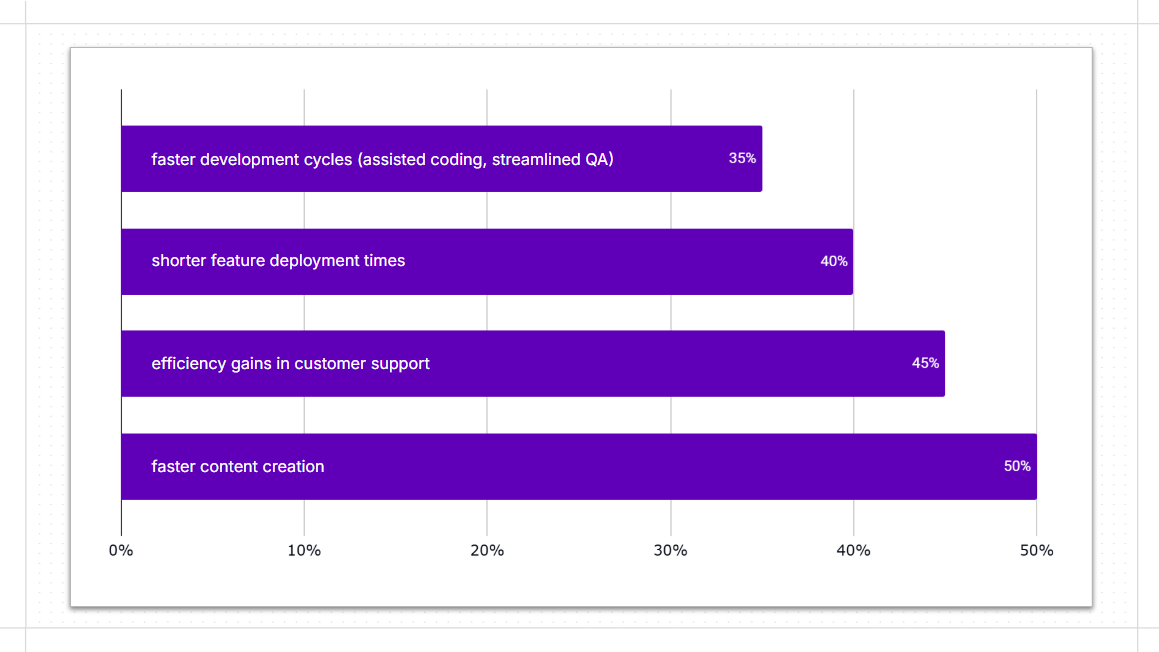
These metrics aren’t just numbers—they’re becoming crucial leverage points for technology leaders seeking to expand their AI initiatives. They provide tangible evidence for securing additional resources and building organizational confidence in AI investments.
Major bottlenecks
However, the path to AI success isn’t without its obstacles. Our research reveals four major bottlenecks that organizations experience and that must be strategically addressed:
- Data readiness: Siloed or the low-quality data availability
- Regulatory complexity: Privacy and compliance hurdles are slowing down progress
- Lack of talent & training: Upskilling your existing teams is vital as outside resources are scarce and often expensive.
- ROI uncertainty and the uncertainty about use cases: Some executives hesitate to move forward without clear benchmarks and no proof of early success and/or do not know where to start.
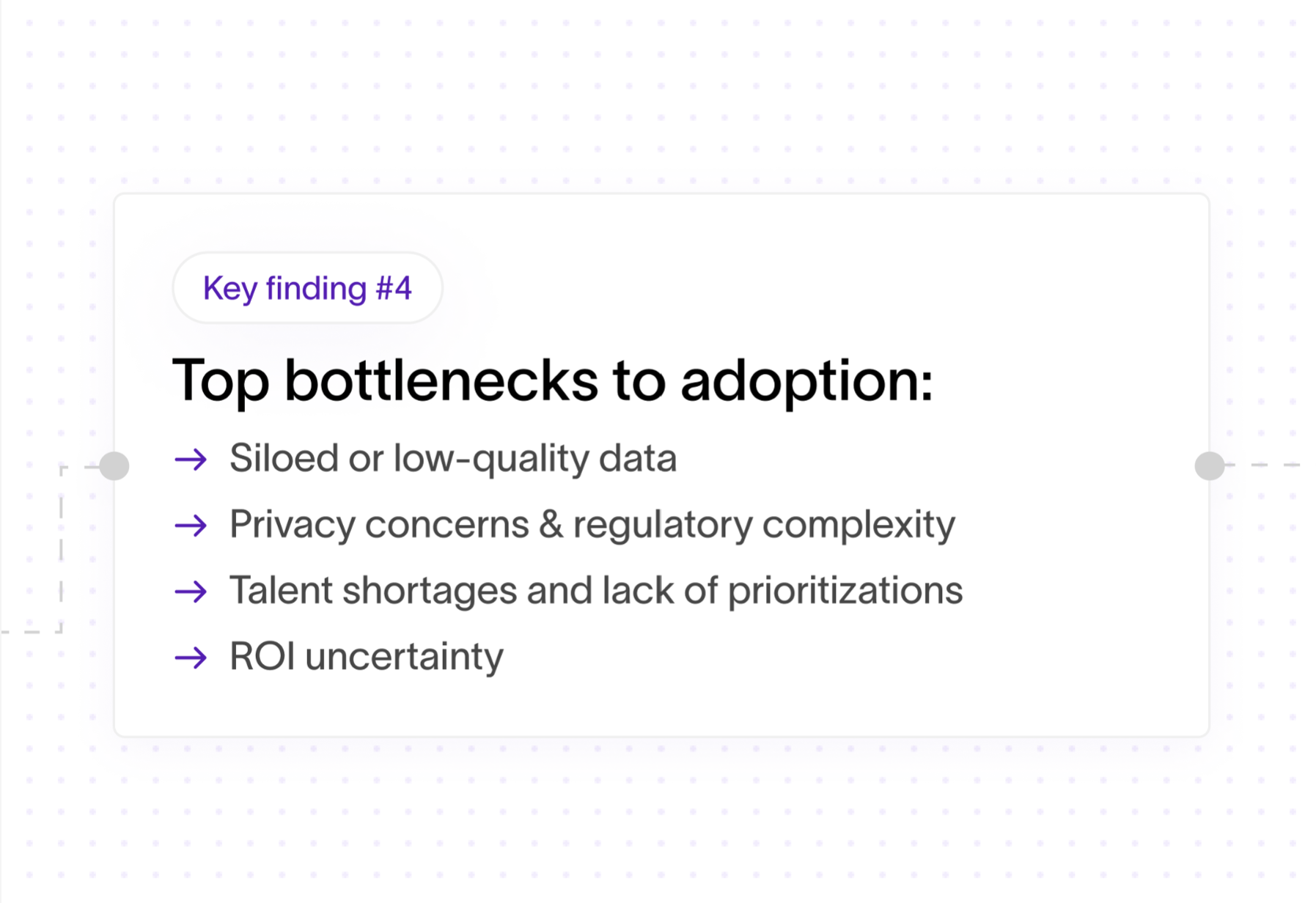
Our findings emphasize however a clear path forward: success in AI implementation demands a balanced approach combining small and focused use cases, iterative development, and robust data foundations.
Tech leaders that have shown success in adoption tell us that the “start small, scale fast” approach has emerged as the overall winning strategy when it comes down to adopting AI. It allows organizations to build momentum on one hand while developing its capabilities and securing larger budgets that are needed for a broader deployment on the other hand.
This evidence-based approach, furthermore, helps organizations to avoid the common pitfall of attempting too much too soon, instead focusing on building a solid foundation for sustainable AI adoption and growth.
3. The AI acceleration roadmap
When looking at the 150+ companies we talked to, we noticed that the ones that had been able to move from one-off experiments to a sustained AI advantage, had followed these steps in one shape or form:
1. It all starts with defining a clear AI vision
Envision how AI could transform your product or service if it were fully integrated into your business. Which workflows become automated? Which user experiences change? From there, choose 1–3 high-value use cases—customer-facing or internal—that align with your broader business goals.
Tip: If you operate in regulated industries, involve compliance early to streamline approvals.
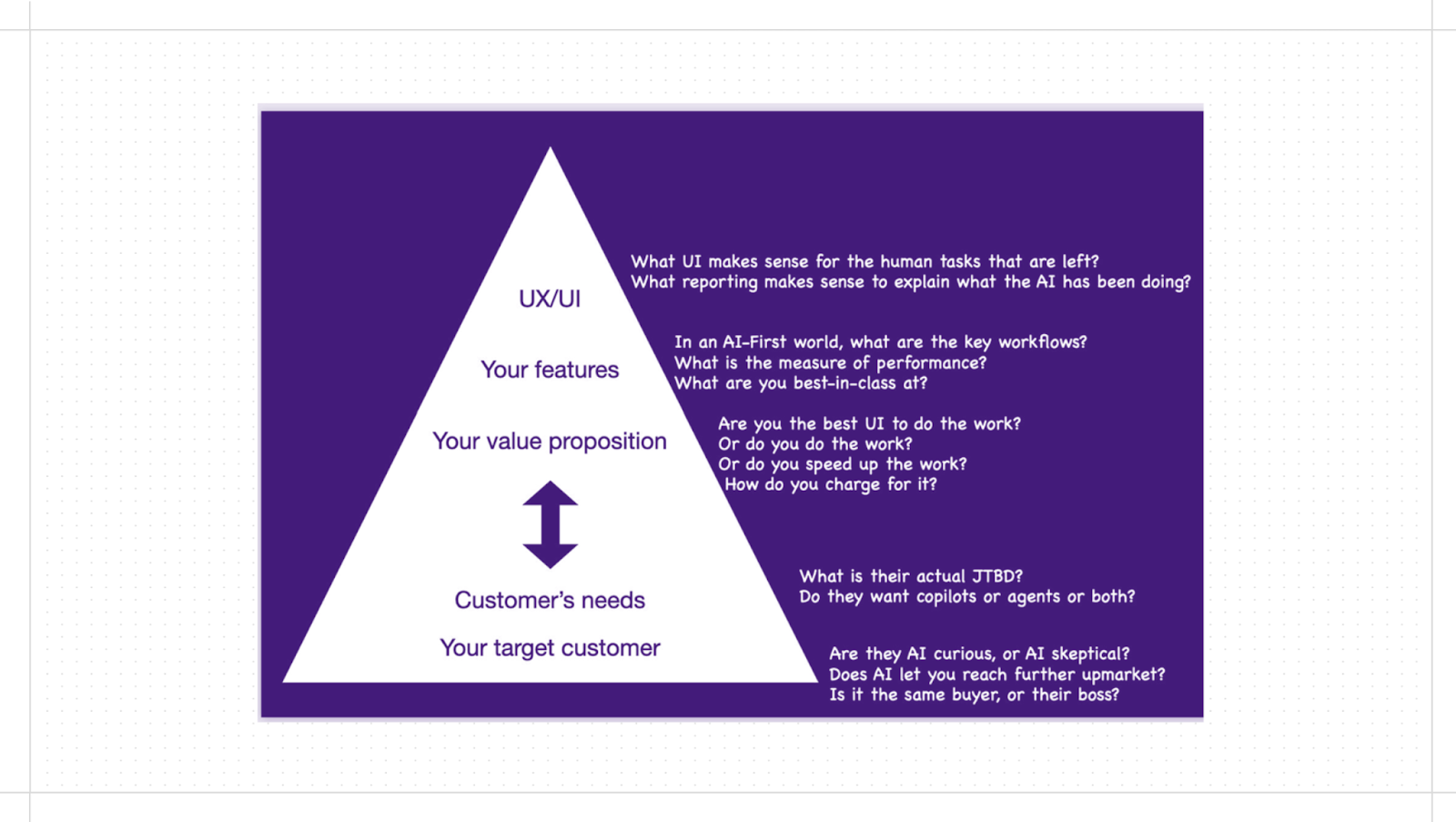
2. Build a focused team
Instead of hiring a fleet of AI PhDs, form a trigger team with existing product owners, developers, and data-savvy staff who know your domain. Offer targeted upskilling on prompt engineering, foundation models, and integration best practices. Also appoint a Product + AI leader to align technical possibilities with user needs and revenue goals.
3. Choose tools & infrastructure
Many companies adopt a multi-vendor approach (OpenAI, Anthropic, or open-source Llama variants) to optimize performance and cost. Invest in robust data pipelines and, if relevant, vector databases for quick retrieval. Start small with the established AI platforms before committing to custom solutions.
Tip: High-quality, well-structured data is often the biggest driver of AI success.
4. Launch pilot projects & iterate
Pick pilot projects that provide actual value but won’t jeopardize core operations if they fall short—e.g., an automated content suggestion feature or a predictive scheduler. Design with human-in-the-loop validation so users can confirm or correct AI outputs. Track key metrics like time saved, revenue uplift, and user satisfaction, then iterate on prompts, data sets, or model configurations to refine performance.
Example: A B2B SaaS platform introduced AI-driven scheduling and saw a 30% drop in manual overhead—which shows proof of value that justified expanding AI to other areas in the business.

5. Scale AI across teams
Once pilots prove their worth, extend AI to internal operations—coding assistants, automated documentation, or a knowledge-base Q&A. Integrate these wins into your main product roadmap so AI stops being an “extra” and becomes fundamental to your offering. Encourage cross-functional collaboration between product, engineering, marketing, and legal to uncover more AI-driven opportunities.
6. Manage compliance & risk
Establish data governance policies on usage, retention, and ethical constraints. Where possible, opt for enterprise-level AI subscriptions with zero data retention and EU hosting. Continuously monitor outputs for errors or bias; disclaimers and moderation mechanisms help to mitigate reputational risks.
Tip: SaaS providers in healthcare or fintech often use private-instance models or keep all processing within EU data centers to meet regulatory needs.
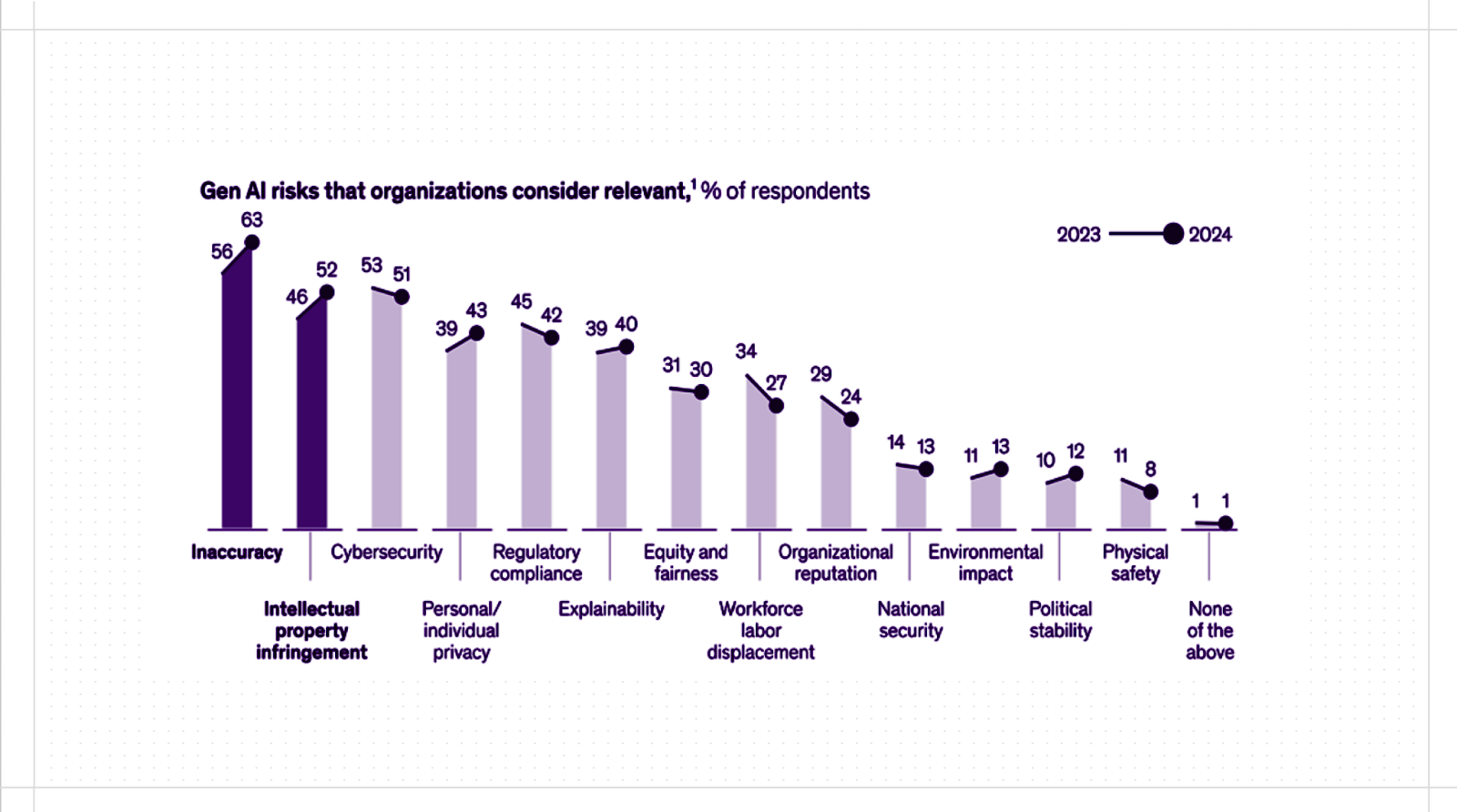
7. Foster a sustainable AI culture
Offer monthly or quarterly training so teams keep pace with AI’s rapid evolution. Encourage employees to share experiments, success stories, and lessons learned with each other. Celebrate quick wins (e.g., better support metrics or faster content creation) to keep the momentum high.
Tip: Some companies form an internal “AI Guild” to coordinate best practices and champion AI adoption across departments.
8. Looking beyond pilots
AI integration is ongoing, so it’s not a one-off project. Successful companies show that they are continuously:
- Refine pilots based on real-world feedback and model improvements
- Explore AI agents that automate entire workflows end-to-end
- Adapt to shifting regulations and emerging toolsets to stay ahead
Following this roadmap and or tips from other entrepreneurs, helps you to move from one-off AI experiments to a sustained AI advantage, delivering measurable business value and forging deeper customer loyalty.

4. Outlook for 2025
The European SaaS landscape truly stands at a defining moment in 2025. AI is fundamentally changing how software companies compete and create value. Understanding the new market dynamics becomes crucial for both the current industry and companies that are now emerging.
Emergence of AI agents & autonomous workflows
AI is set to evolve from just “smart features” to autonomous agents that are capable of handling end-to-end processes. For example:
- Customer support: AI agents will handle complete issue resolution, including the proactive follow-up.
- Sales & marketing: Expect AI-driven lead qualification, automatic email campaigns, and even active pipeline management.
- DevOps & QA: We will see even more automated code generation, continuous testing, and performance monitoring with minimal human oversight.
Talent availability & squads
The scarcity of specialized ML talent will gradually ease as more developers learn to use pretrained models and low-code AI frameworks.
- Cross-functional “AI squads”: We expect that your current product managers that have an interest in AI, your data-savvy developers, and your UX specialists start to form (virtual) AI squads that will drive your AI features development.
- AI centers of excellence: These Squads will form internal guilds or committees that together will standardize practices, share knowledge, and/or address ethical or compliance concerns.
Regulatory landscape & ethical AI
Stricter regulations—like the anticipated EU AI Act—are likely to formalize and set the standards for explainability, bias auditing, and data protection. Rather than just hindering progress, these rules can also serve as:
- Differentiators: Companies that are able to master this sort of “compliant AI” will gain the trust of large enterprises and government entities.
- Catalysts for innovation: Building privacy and security into AI solutions from the ground up can unlock new market segments that demand transparent and responsible tech.
Beyond hype: practical value creation
This year we expect that AI will be less about flashy demos and fun “magic wand” type features, and more about sustained business impact.
- Deeper integration: AI is going to be woven directly into core user experiences, not just used as add-on features.
- Expanded revenue streams: From standalone AI modules to premium “AI-as-a-Service” offerings.
For European SaaS, 2025 marks a tipping point: those that integrate AI responsibly and comprehensively will establish lasting competitive advantages, while laggards may find it increasingly difficult to catch up. The time to hold out and see what happens is behind us.
5. A Call to arms: seizing Europe’s AI opportunity
We are standing at a crossroad now. AI has evolved from an interesting experiment to a core capability that can drive growth, efficiency, and innovation. While Europe trails the US in sheer adoption speed, its strengths in privacy, compliance, and trusted technology set the stage for differentiated AI leadership.
Bridging the gap
Most organizations grasp AI’s potential but remain mired in endless pilots or disjointed strategies. The path forward requires focus on high-impact use cases and quick ROI, upskilling existing teams, and integrating AI into the core product roadmap and internal organisation —not as a novelty, but as a defining value proposition.
Preparing for 2025 and beyond
By 2025, AI will likely power everything from customer interactions to DevOps pipelines, demanding a culture of continuous improvement, robust data governance, and ethical diligence. Those who invest now—resolving compliance hurdles, turning pilot projects into production features, and nurturing an AI-savvy workforce—will define the next wave of European SaaS champions.
So, some core steps to take now
- Secure quick wins: Target pilots that address urgent user pain points or streamline internal processes to build momentum.
- Embed AI everywhere you can: Align AI initiatives with your central product strategy, fostering cross-functional ownership and collaboration.
- Double down on governance: Reinforce privacy, data protection, and responsible AI development to win customer trust and compliance-sensitive markets.
- Adapt constantly: AI evolves rapidly; keep your organization agile by investing in ongoing training, iterative pilot feedback, and flexible tech stacks.
With thoughtful planning, Europe’s regulatory and ethical emphasis can become its competitive advantage, fueling a new generation of AI-enabled SaaS solutions that resonate globally. This is a call to arms for CIOs, CPOs, and CTOs: invest decisively and deliver tangible value—before the window of opportunity narrows.