AI-Powered Knowledge Bases: The Secret Weapon Smart Businesses Are Using in 2025
Discover how AI-powered knowledge bases eliminate the paradox of information overload yet knowledge scarcity, saving employees 8-10 hours monthly and delivering measurable ROI across departments by providing intelligent, contextual answers instead of mere search results.
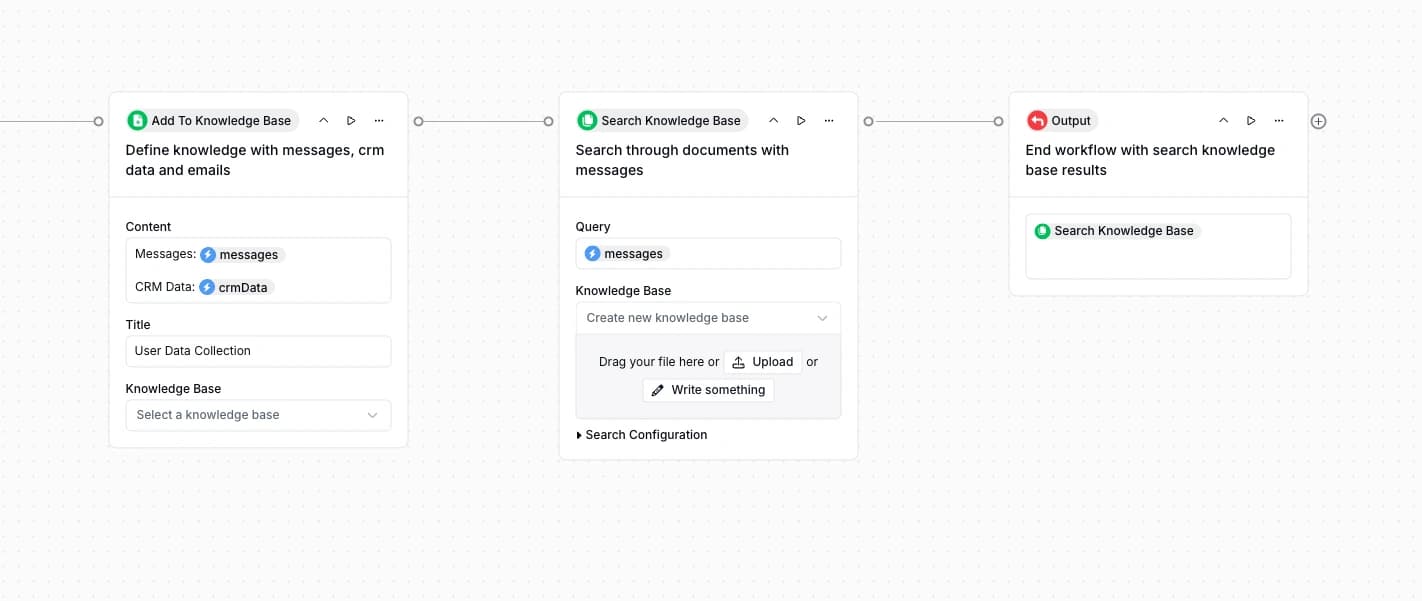
Most companies are drowning in information while simultaneously starving for knowledge.
It's the classic corporate paradox: terabytes of documents, emails, messages, and meeting notes... yet when someone needs a specific answer, it feels like searching for a digital needle in a haystack.
And the cost? According to McKinsey, employees spend nearly 20% of their workweek just hunting for information. That's a full day every week just trying to find stuff that already exists somewhere in your organization.
This is where AI-powered knowledge bases come in - not just as a nice-to-have tech toy, but as a business-critical system that's reshaping how companies operate in 2025.
What exactly is an AI-powered knowledge base?
Let's cut through the jargon. An AI-powered knowledge base isn't just a fancy search bar slapped onto a document repository.
It's an intelligent system that:
- Connects to all your information sources - documents, emails, messages (Slack, Teams, etc.), wikis, databases, ticketing systems, you name it
- Understands context and relationships between different pieces of information
- Delivers answers, not just results - synthesizing information rather than just pointing to it
- Learns continuously from user interactions and feedback
- Speaks human - you can ask natural questions and get comprehensive answers
Think of traditional knowledge bases as libraries with card catalogs - you still need to know what you're looking for and where to find it. AI knowledge bases are more like having a subject matter expert who's read everything in the library and can have a conversation about any topic.
The core technologies making this possible include:
- Natural Language Processing (NLP) to understand human questions
- Vector databases that organize information based on meaning rather than keywords
- Retrieval Augmented Generation (RAG) that pulls relevant info before generating answers
- Conversation memory to maintain context through multiple exchanges
The problem with these technologies: you need an "ai-native" builder to build and maintain knowledge bases like this in a couple of clicks, or you need to hire a bunch of AI engineers.
Why traditional knowledge bases just don't cut it anymore
The old-school knowledge base is dying, and honestly, it's about time.
Here's why they're falling behind:
Nobody uses them. Traditional knowledge bases require manual maintenance - creating categories, tagging content, writing summaries. It's administrative overhead that nobody has time for, so they quickly become outdated.
Search is broken. Keyword-based search engines miss context and relationships between documents. Type "pricing policy" and you'll find every document containing those words, but not necessarily the answer you need.
Information silos are everywhere. Your company's knowledge isn't just in one system - it's fragmented across dozens of tools, and traditional knowledge bases can't bridge these gaps effectively.
Scale problems. As content grows, navigation becomes more complex, and findability decreases. The larger your traditional knowledge base gets, the less useful it becomes.
Look at what happened to WebMD's traffic after ChatGPT launched - they're down 40% since then. When direct answers are available through AI, nobody wants to dig through articles manually.
The business case: Why you need an AI knowledge base yesterday
The ROI on AI knowledge bases isn't theoretical - it's immediate and measurable:
- Time reclaimed: Employees gain back 8-10 hours per month previously lost to searching for information.
- Knowledge retention: When senior employees leave, their institutional knowledge doesn't walk out the door with them.
- Onboarding acceleration: New hires become productive 40% faster when they can instantly access tribal knowledge.
- Support ticket reduction: Companies report 30-50% fewer internal support tickets when employees can self-serve answers.
- Consistency in communication: Customer-facing teams deliver accurate, consistent information to customers.
One of our clients estimated they save $2.4M annually just from the improved productivity their AI knowledge base delivers. Their calculation was straightforward:
1,000 employees × 8 hours saved monthly × $25/hour × 12 months = $2.4M
And that doesn't even account for improved decision quality, reduced training costs, and faster time-to-market.
How different departments benefit from AI knowledge bases
AI knowledge bases aren't a one-size-fits-all solution - they deliver specific value to different teams:
Customer Support
- Instant access to product information, troubleshooting steps, and policy details
- 70% faster resolution times
- Consistent answers across support channels
- Training new agents in days instead of weeks
Sales
- Real-time competitive intelligence during calls
- Quick access to case studies and social proof
- Accurate product details and pricing information
- New hire ramp-up time cut in half
Product & Engineering
- Code documentation and architecture decisions at fingertips
- API specifications and integration guides
- Previous discussions about features and roadmap
- Bug history and resolution approaches
HR & Operations
- Policy questions answered immediately
- Consistent interpretation of company procedures
- New hire questions handled without manual intervention
- Process documentation available on demand
The pattern is clear: every department spends too much time searching for information they already have. AI knowledge bases eliminate this waste.
Implementation guide: Getting started without the headaches
Getting an AI knowledge base up and running doesn't have to be a 6-month IT project. Here's a practical approach that works:
1. Start with a specific use case
Don't boil the ocean. Pick one team or problem area with:
- High-value knowledge needs
- Frequent, repetitive questions
- Measurable outcomes
Support teams are often a great starting point, as they have clear metrics around resolution time and ticket volume.
2. Identify and connect your knowledge sources
Take inventory of where your important information lives:
- Documentation systems (Confluence, Notion, Google Docs)
- Communication platforms (Slack, Teams)
- Ticket systems (Zendesk, Jira)
- Wikis and intranets
- Shared drives and repositories
Modern AI knowledge base platforms like Lleverage provide ready-made connectors to ingest data from these common sources, making setup straightforward.
3. Configure your AI and test extensively
The quality of answers depends on:
- Using the right base models
- Proper prompt engineering
- Clear guidelines for the AI
- Testing with real user questions
This is where having a no-code platform makes a huge difference. You shouldn't need ML engineers to get good results.
4. Roll out incrementally with feedback loops
Start with a small user group, gather feedback, and iterate:
- What questions aren't getting good answers?
- Which information sources might be missing?
- Are there opportunities to improve the AI's responses?
Once you're seeing consistently good results, expand to more users.
5. Measure and expand
Track concrete metrics:
- Usage patterns
- Response quality ratings
- Time saved
- Impact on key performance indicators
Use these insights to expand to additional use cases and departments.
Common objections (and why they don't hold water)
Let's address the elephants in the room:
"Our data is too sensitive for AI"
Modern AI knowledge base solutions offer enterprise-grade security, including:
- Zero data retention by the underlying models
- Private cloud or on-premise deployment options
- Encrypted data storage and transmission
- Role-based access controls
- Audit logging and compliance features
Unlike consumer AI tools, enterprise solutions are built with security-first architectures.
"We don't have clean, organized data"
That's precisely the point. AI knowledge bases excel at making sense of messy, unstructured information. They can:
- Extract relevant content from lengthy documents
- Understand the relationships between disorganized files
- Identify the most trustworthy sources
- Improve over time through usage and feedback
The messier your data, the more value an AI system provides.
"Our teams won't adopt another tool"
The best AI knowledge bases integrate where your employees already work:
- Slack and Teams plugins
- Browser extensions
- API access for custom integration
- Mobile apps for on-the-go access
When answers are available without switching context, adoption happens naturally.
"It's just another tech fad"
Knowledge management isn't new - organizations have struggled with it for decades. What's changed is the technology's capability to deliver on the promise. AI knowledge bases solve fundamental business problems that have existed since the dawn of the information age.
The future: Where AI knowledge bases are heading
The knowledge base space is evolving rapidly, with several trends emerging:
- Multimodal capabilities - Processing and understanding images, diagrams, and video content alongside text
- Proactive knowledge delivery - AI that anticipates information needs based on context and delivers relevant knowledge before it's requested
- Knowledge graphs - Visualizing relationships between information to uncover insights that might otherwise remain hidden
- Personalized knowledge delivery - Tailoring information based on the user's role, expertise level, and past interactions
- AI agents - Moving beyond answering questions to taking actions based on the knowledge base
The line between knowledge bases and intelligent assistants is blurring, creating systems that not only know things but can use that knowledge to perform tasks.
FAQs about AI-powered knowledge bases
How is an AI knowledge base different from just using ChatGPT?
General-purpose AI like ChatGPT lacks context about your specific business. It doesn't have access to your internal documents, policies, or historical decisions. An AI knowledge base is trained on your company's information, ensuring accurate, contextual answers based on your actual data.
Do we need data scientists to implement and maintain an AI knowledge base?
Not anymore. Modern platforms like Lleverage offer no-code interfaces for setting up and managing AI knowledge bases. Non-technical users can connect data sources, configure the AI, and monitor performance without specialized ML expertise.
What happens when information changes or gets updated?
Good AI knowledge base systems continuously synchronize with your data sources, ensuring they always have the latest information. When documents are updated, the knowledge base reflects those changes automatically.
How do we ensure the AI gives accurate answers?
Three key factors ensure accuracy:
- Connecting comprehensive, up-to-date information sources
- Using retrieval techniques that ground the AI's responses in your actual documents
- Implementing feedback mechanisms so users can flag incorrect responses
Some platforms also offer human review workflows for sensitive topics.
What kind of ROI can we expect?
While results vary by organization, typical outcomes include:
- 20-30% reduction in time spent searching for information
- 25-40% faster onboarding for new employees
- 30-50% reduction in internal support tickets
- Significant improvement in employee satisfaction scores
Most companies see positive ROI within 3-6 months of implementation.
The knowledge in your organization represents years of experience, countless hours of work, and millions in investment. An AI-powered knowledge base ensures that intellectual capital doesn't just exist somewhere in your systems—it becomes accessible, usable, and valuable to every employee, every day.
Ready to explore how an AI knowledge base could transform your organization? Book a demo with our team to see Lleverage's AI knowledge base solution in action.